Elicit and AI Safety
The two main impacts of Elicit on AI Safety are improving epistemics and pioneering process supervision.
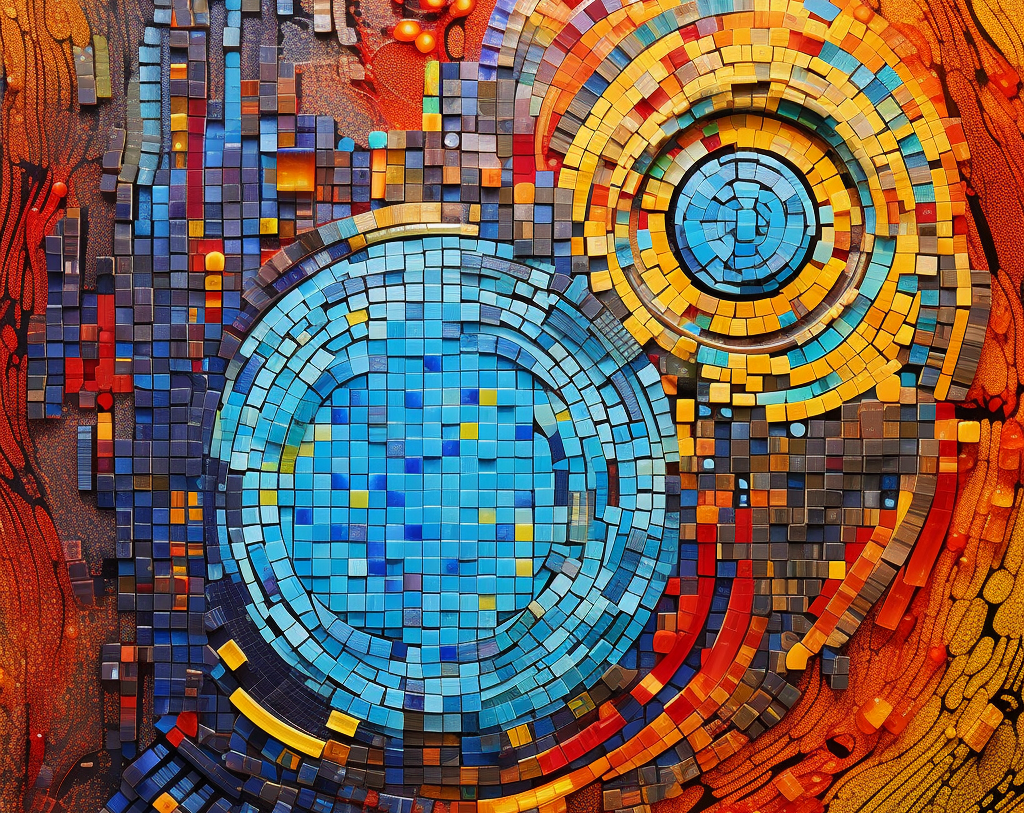
Improving epistemics
As AI becomes a bigger deal, the world will become more complex, things will change more quickly, and some information will become less trustworthy. In that world, it is increasingly important that AI can help guide key decisions.
This includes narrowly supporting technical alignment research, but also applies to improving AI policy and coordination, all the way to helping people behave more wisely in general, reducing human-generated risk across the board.
For high-impact situations, it's especially important that the reasoning is correct, and that the systems we're deploying don't themselves introduce further risks. To avoid introducing risk, Elicit is built using process supervision. We're building infrastructure that will let us orchestrate millions of AI workers for the most important knowledge & decision-making tasks in ways that are systematic, transparent, and unbounded.
Over time, Elicit will become a general-purpose tool for research and reasoning that can provide the most reliable answers for the most important questions.
Pioneering process supervision
At Elicit, we favor compositional, transparent systems built out of bounded components over large black-box models. We're building these process-based systems on human-understandable task decompositions, with direct supervision of reasoning steps.
We will demonstrate the ideal deployment of process-based systems in the world to users, companies, and foundation model labs, so that:
- Users know that they can demand transparency and control from the language model products they use
- Companies know how to meet these needs, and know about the dangers of excessive outcome focus
- Foundation model labs in turn support these startups and users
We'll build on the work our team has already done on factored cognition and task decomposition.
The future doesn't have to look like increasingly giant hard-to-align black-box models. Our bet is that a mix of clear demonstrations, regulation, self-regulation, and reasoning about the pros & cons of different approaches to AI deployment will lead to a future where process-based systems dominate.